Intelligence requires the whole brain
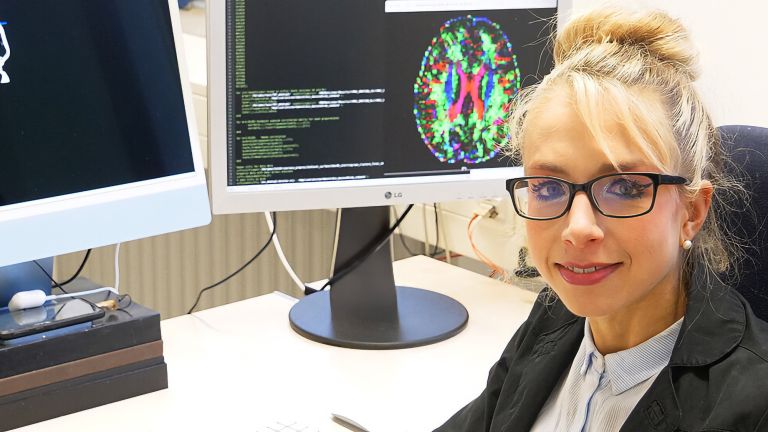
A team of Würzburg neuroscientists investigates communication pathways in the brain and predicts intelligence. A new study approach uses machine learning to improve our conceptual understanding of intelligence.
Published: 11.12.2024
The human brain is the central control organ of our body. It processes sensory information and enables us, among other things, to form thoughts, make decisions and store knowledge. With how much our brain is capable of, it seems almost paradoxical how little we still know about it.
Jonas Thiele and Dr. Kirsten Hilger, head of the “Networks of Behavior and Cognition” research group at the Department of Psychology I at Julius-Maximilians-Universität Würzburg (JMU), are among those who are on the trail of the most complex and complicated organ. Their latest study was recently published in the scientific journal PNAS Nexus: “Choosing explanation over performance: Insights from machine learning-based prediction of human intelligence from brain connectivity.”
The researchers used data sets from a large-scale data sharing project based in the US: the Human Connectome Project. With the help of fMRI – an imaging method that measures changes in brain activity – over 800 people were examined. Both at rest and while they performed various tasks.
The Würzburg-led team looked at various connections that map the communication strength between different brain regions and made predictions about individual intelligence scores based on these observations.
“Many studies predicting intelligence from brain connections have been published in the last years and they also achieve quite good predictive performance,” says Kirsten Hilger. However, the neuroscientists question their deeper meaning, as the predictions would never be as accurate as the results of an intelligence test. “We therefore intended to move away from the pure prediction of intelligence scores and instead better understand the fundamental processes in the brain. We hope that this will give us a better understanding of the neural code of individual differences in intelligence.”
Kirsten Hilger hopes that colleagues will follow her example and that more studies will be designed in the future aiming at improving the conceptual understanding of human cognition with a focus on interpretability.
Funktionelle Magnetresonanztomographie
Funktionelle Magnetresonanztomographie/-/functional magnetic resonance imaging
Eine Modifikation der Magnetresonanztomographie oder –tomografie (MRT, englisch MRI) die die Messung des regionalen Körperstoffwechsels erlaubt. In der Hirnforschung wird besonders häufig der BOLD-Kontrast (blood oxygen level dependent) verwendet, der das unterschiedliche magnetische Verhalten sauerstoffreichen und sauerstoffarmen Bluts nutzt. Ein hoher Sauerstoffverbrauch kann mit erhöhter Aktivität korreliert werden. fMRT-Messungen haben eine gute räumliche Auflösung und erlauben so detaillierte Information über die Aktivität eines bestimmten Areals im Gehirn.
Three Types of Intelligence
The team distinguished three types of intelligence in their predictions: Fluid intelligence refers to the ability to solve logical problems, recognize patterns and process new information, independent of existing knowledge or learned skills.
Crystallized intelligence encompasses the knowledge and skills that a person acquires over the course of their life. This includes general knowledge, experience and understanding of language and concepts. It arises through education and experience.
Together, these two forms make up general intelligence. The best predictive performance was achieved with general intelligence, followed by crystallized and fluid intelligence.
Brain-wide Connections Best Predict Intelligence
Various theoretical considerations determined which different connections in the brain were examined. In addition, randomly selected connections were also tested. One crucial observation: the distribution of connections across the entire brain as well as the pure number of connections were most important for predictive performance, more important than between which exact brain regions the individual connections were located.
“The interchangeability of the selected connections suggests that intelligence is a global property of the whole brain. We were able to predict intelligence not just from a specific set of brain connections, but from different combinations of connections distributed throughout the brain,” says Hilger.
Results Outperform Established Theories
While established theories of intelligence often focus on specific areas of the brain - such as the prefrontal cortex - the results of the study suggest that connections between additional brain regions are important for intelligence: “The connections of brain regions proposed in the most popular neurocognitive models of intelligence produced better results than randomly selected connections. However, the results were even better when complementary connections were added,” reports Kirsten Hilger.
Overall, this suggests that there are even more aspects of intelligence than previously assumed that are waiting to be understood in future.
Original Publication
Jonas A Thiele, Joshua Faskowitz, Olaf Sporns, Kirsten Hilger, Choosing explanation over performance: Insights from machine learning-based prediction of human intelligence from brain connectivity, PNAS Nexus, Volume 3, Issue 12, December 2024, pgae519, DOI: https://doi.org/10.1093/pnasnexus/pgae519